Table of Contents
ToggleHealthcare Data Management
Definition and Importance
Healthcare data management refers to the systematic organization, storage, and analysis of data related to patient care and health services. It plays a pivotal role in modern healthcare as it helps in improving treatment outcomes, enhancing efficiency, reducing operational costs, and facilitating compliance with regulatory standards. Effective data management is essential for healthcare providers to access accurate and timely information, which is crucial for decision-making processes that impact patient care directly.
Evolution of Healthcare Data Management
The evolution of Healthcare Data Management is marked by significant milestones from paper-based records to advanced electronic health records (EHRs), big data analytics, and the integration of AI technologies. Initially, healthcare data was primarily stored in physical files, limiting access and analysis capabilities. Over the years, the shift to digital records has enabled more complex analyses and real-time data accessibility. Today, the field is transitioning towards more integrated systems that leverage cloud computing and AI to provide more comprehensive and proactive healthcare solutions.
Key Components of Healthcare Data Systems
The key components of healthcare data systems include:
- Data Warehousing: Centralized repositories where data from various sources is stored and managed.
- Data Mining: Techniques used to explore large datasets to discover patterns and relationships.
- Data Security: Measures and protocols to protect data from unauthorized access and breaches.
- Interoperability: The ability of different IT systems and software applications to communicate, exchange, and use the data cohesively.
Data Collection in Healthcare

Sources of Healthcare Data
Healthcare data comes from a variety of sources including:
- Clinical data: From electronic health records (EHRs), medical imaging, genetic information, and laboratory results.
- Patient-generated data: From wearable technologies, mobile apps, and patient-reported outcomes.
- Administrative data: Billing records, claims data, and scheduling systems.
Techniques for Data Collection
Techniques for collecting healthcare data vary widely but commonly include:
- Electronic data capture (EDC): Using software to collect clinical trial data electronically.
- Patient monitoring systems: Continuous data collection from medical devices.
- Surveys and questionnaires: Directly from patients to gather subjective data about health status and treatment outcomes.
Challenges and Best Practices
Challenges in Healthcare Data Management and collection include ensuring data quality, managing the sheer volume of data, and protecting patient privacy. Best practices to address these challenges include:
- Implementing robust data governance frameworks to ensure data accuracy and consistency.
- Using advanced data encryption and security measures to protect data privacy.
- Employing interoperable data systems that can communicate seamlessly with other systems to enhance data utility across different healthcare providers.
Data Storage Solutions

Traditional vs. Modern Storage Systems
The landscape of data storage in healthcare has evolved significantly from traditional systems to modern solutions. Traditional storage systems typically involve on-site data centers and physical servers, which often require substantial space and energy, along with intensive management and maintenance. In contrast, modern storage solutions, like cloud storage and hybrid systems, offer scalability, flexibility, and accessibility. They allow healthcare providers to store large volumes of data without the physical footprint of traditional systems and facilitate quick access to data for healthcare professionals, regardless of their location.
Cloud Storage and its Advantages
Cloud storage has become increasingly popular in Healthcare Data Management due to its numerous advantages. It provides scalability, allowing healthcare facilities to easily adjust their storage space based on current needs, and cost-effectiveness, as it eliminates the need for large capital investments in physical infrastructure. Additionally, cloud storage enhances collaboration among healthcare professionals by enabling seamless data sharing across different geographic locations. This accessibility improves the continuity of care and supports more timely and informed decision-making in patient care.
Data Security and Compliance
With the transition to digital storage systems, data security and compliance have become paramount in healthcare. Healthcare organizations must adhere to stringent regulations, such as HIPAA in the U.S., which sets standards for the protection of sensitive patient data. Ensuring data security involves implementing advanced encryption methods, regular security audits, and compliance checks. Furthermore, healthcare entities must establish robust data breach response plans to mitigate any potential risks associated with data storage and maintain the trust of patients and stakeholders.
Data Integration Techniques
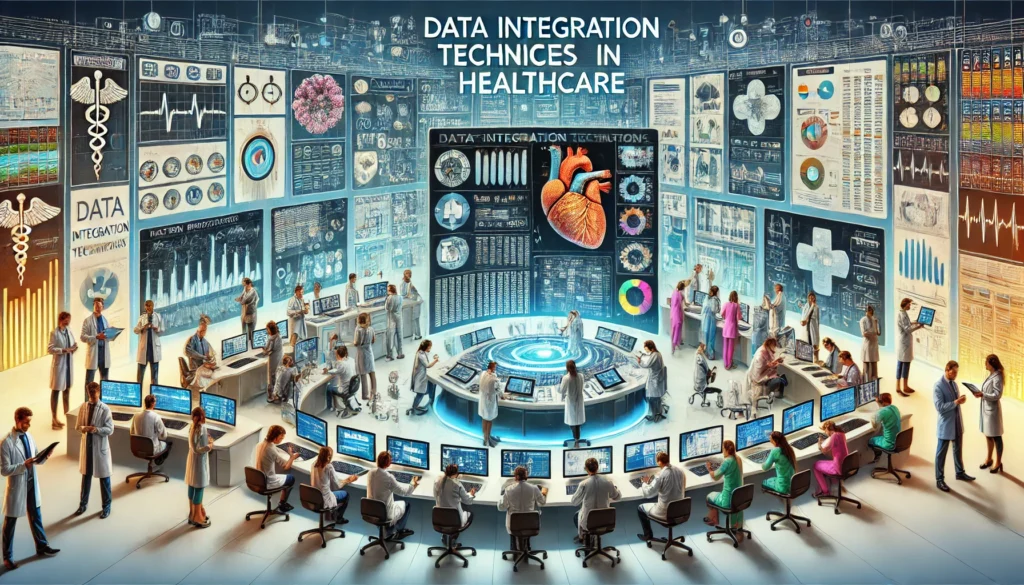
Importance of Interoperability
Interoperability in healthcare is critical for the effective exchange and utilization of patient information across different healthcare systems and applications. It supports comprehensive patient care by enabling various healthcare providers to access and understand the shared data seamlessly. Improved interoperability leads to better patient outcomes, as it ensures that patient data is complete, accurate, and available when needed, thus facilitating informed clinical decisions.
Common Data Integration Models
Several data integration models are used in healthcare to facilitate the efficient merging of data from various sources. The most common models include:
- Consolidated Data Warehousing: This model involves collecting data from different sources and storing it in a central warehouse where it can be accessed and analyzed collectively.
- Federated Database System: Unlike data warehousing, federated databases allow organizations to leave data in its original location, integrating it virtually for querying and analysis without duplication.
- Data Lakes: A data lake stores vast amounts of raw data in its native format. This model is flexible and scalable, suitable for healthcare organizations that generate and collect massive volumes of diverse data types.
Overcoming Integration Challenges
Data integration in healthcare faces several challenges, including data heterogeneity, privacy concerns, and technical incompatibilities between different systems. To overcome these challenges, healthcare organizations can employ strategies such as adopting standards for data exchange (e.g., HL7, FHIR), using middleware to facilitate communication between disparate systems and leveraging APIs for smoother data access and integration. Additionally, ongoing training and development of IT staff are crucial for adapting to evolving integration technologies and maintaining system compatibility over time.
Data Analysis and Reporting of Healthcare Data Management
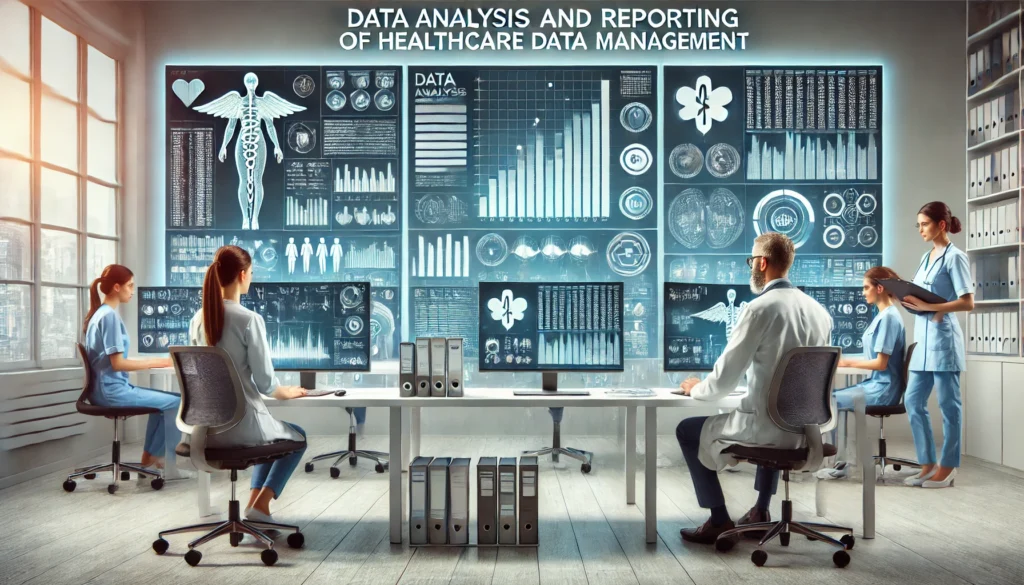
Tools for Data Analysis in Healthcare
In the realm of healthcare, data analysis tools range from basic software for statistical analysis to advanced platforms that incorporate machine learning algorithms. Tools like SAS, SPSS, and R are widely used for statistical testing and data exploration. More sophisticated systems like Tableau and Microsoft Power BI enable healthcare organizations to visualize data trends and patterns effectively, facilitating better strategic decisions. The selection of tools often depends on the specific needs of the healthcare entity, including considerations like data volume, complexity, and the required granularity of analysis.
Generating Reports: Practices and Purposes
Generating reports in healthcare serves multiple purposes: from regulatory compliance and monitoring treatment outcomes to optimizing operational efficiency and enhancing patient care. Effective reporting practices involve the accurate and timely generation of reports to ensure that decision-makers have the most relevant and current data at their fingertips. Automated reporting systems are increasingly becoming standard, reducing human error and freeing up resources for more critical tasks, like patient care.
Case Studies: Effective Use of Data Analytics
Several case studies highlight the transformative power of effective data analytics in healthcare. For example, a major hospital could use data analytics to reduce patient wait times by predicting peak hours and staffing accordingly. Another case could involve a health system using analytics to monitor and improve the quality of care for chronic disease patients by tracking adherence to treatment protocols and outcomes across its network.
Role of Artificial Intelligence in Healthcare Data Management
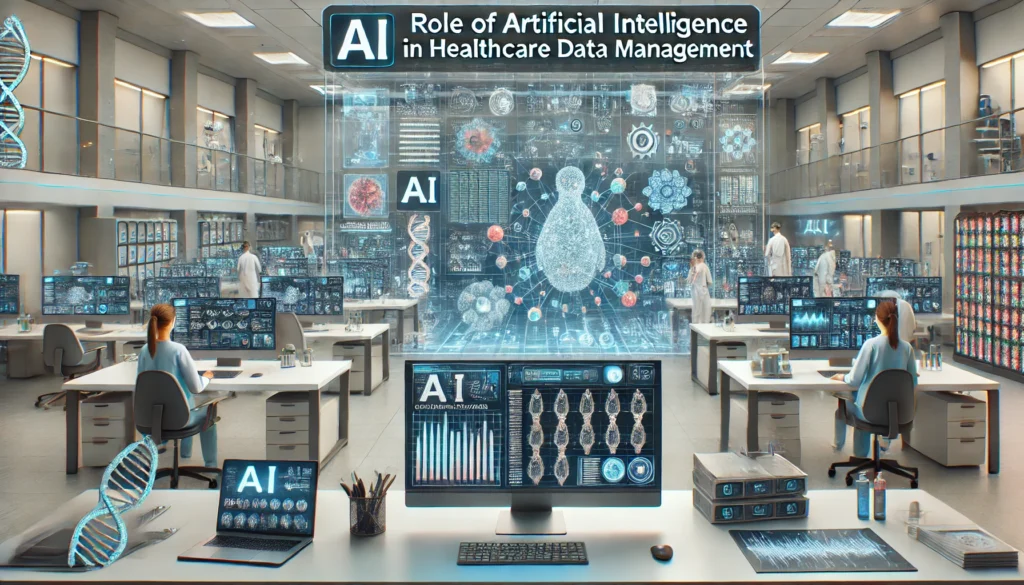
AI in Data Processing and Analysis
Artificial Intelligence (AI) has revolutionized the way data is processed and analyzed in healthcare. AI algorithms can handle large volumes of data far more quickly and accurately than human analysts, identifying patterns and anomalies that might go unnoticed otherwise. For instance, AI systems can analyze imaging data to assist in the early diagnosis of diseases like cancer, often with greater accuracy than traditional methods. AI also helps in automating routine data processing tasks, allowing healthcare providers to focus more on patient care.
Predictive Analytics and Patient Care
Predictive analytics in healthcare utilizes AI to forecast outcomes based on data trends. This capability is particularly useful in managing chronic conditions, where predictive models can indicate potential health deteriorations before they occur, allowing for preemptive intervention. Such analytics can also predict patient inflow in hospitals, helping to better manage resources like beds and medical staff to enhance operational efficiency.
Ethical Considerations and AI
The integration of AI in healthcare data management brings forth significant ethical considerations. Issues such as data privacy, consent, and bias in AI algorithms are at the forefront of discussions. Ensuring that AI systems are transparent and accountable is crucial to maintaining trust among patients and practitioners. Moreover, there is a growing need to develop guidelines and regulations that ensure AI is used responsibly and ethically in healthcare settings.
Blockchain Technology in Healthcare Data Management
Blockchain for Data Security
Blockchain technology is revolutionizing the way data security is approached in healthcare. By leveraging a decentralized ledger system, it ensures that data is immutable and tamper-proof. Each transaction or data entry is recorded as a block and chained to previous entries, creating a secure and transparent audit trail. This method significantly reduces the risk of unauthorized access and data breaches, which are common in traditional centralized data storage systems.
Applications in Data Integrity and Access
The integrity of medical data is crucial for accurate diagnosis and treatment. Blockchain enhances data integrity by maintaining a consistent, unalterable record of all patient data, accessible by authorized personnel only. It also facilitates better access to data across different healthcare providers, ensuring that patients receive consistent and informed care, regardless of location. For example, a blockchain system can enable real-time access to patient histories, lab results, and treatment plans, improving coordination and outcomes.
Limitations and Future Prospects
Despite its potential, blockchain technology in healthcare faces several limitations. The high cost of implementation, the complexity of integrating with existing systems, and scalability issues are significant barriers. Additionally, there are concerns about the speed of transactions on blockchain networks, which can be slower than traditional databases due to the nature of consensus mechanisms. Looking forward, advancements in blockchain technology may address these issues, leading to wider adoption in healthcare systems. Researchers and developers are exploring hybrid models that combine the strengths of blockchain with other technologies to create more efficient, scalable systems.
Regulatory Compliance and Healthcare Data Management
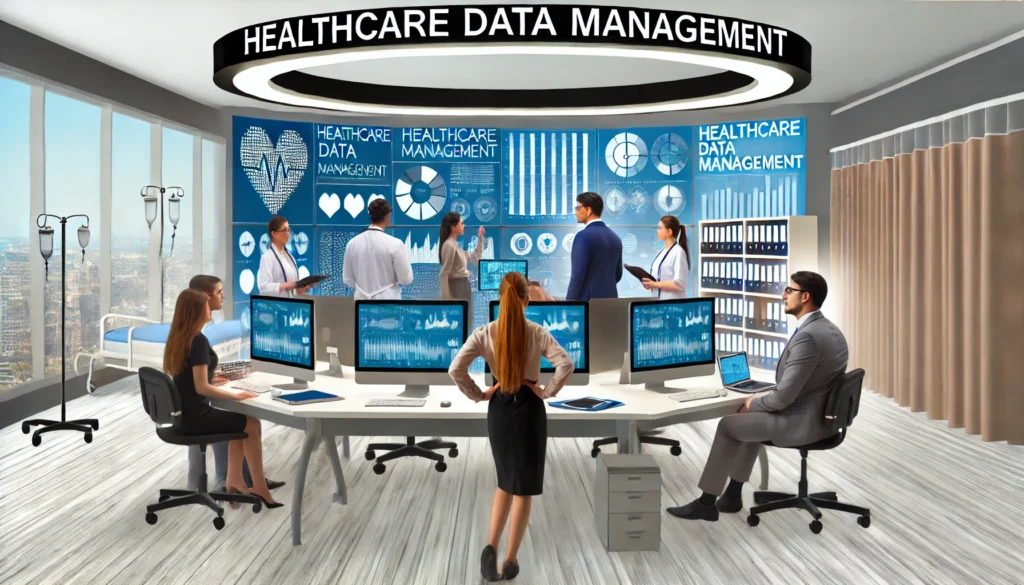
Understanding HIPAA and GDPR
Regulatory frameworks like the Health Insurance Portability and Accountability Act (HIPAA) in the U.S. and the General Data Protection Regulation (GDPR) in the EU play critical roles in healthcare data management. HIPAA sets the standard for protecting sensitive patient data, while GDPR enhances data protection and privacy for all individuals within the European Union. Healthcare organizations must understand the specific requirements and provisions of these regulations to ensure compliance and avoid substantial penalties.
Impact on Healthcare Data Management Practices
The requirements of HIPAA and GDPR have a profound impact on data management practices within healthcare organizations. These regulations mandate rigorous data protection measures, regular audits, and the need for explicit patient consent for data usage. As a result, healthcare entities must adopt comprehensive data management systems that not only comply with these regulations but also provide adequate security measures to protect patient information from breaches and unauthorized access.
Navigating Global Compliance Challenges
Global healthcare organizations face additional challenges when managing data across different regulatory environments. They must navigate varying compliance requirements in each country where they operate, which can complicate data management strategies. Best practices include implementing universal data protection standards that meet the highest regulatory requirements globally and employing legal experts to ensure ongoing compliance with local and international laws. This approach helps mitigate risks and ensures that patient data is managed responsibly and ethically across borders.
Future Trends in Healthcare Data Management

Emerging Technologies and Their Impact
As we move forward, emerging technologies are set to revolutionize the landscape of healthcare data management. Artificial intelligence (AI), machine learning, and blockchain are at the forefront, offering unprecedented capabilities in data processing and security. AI and machine learning algorithms can predict patient outcomes, personalize treatment plans, and optimize resource allocation based on real-time data analysis. Meanwhile, blockchain technology promises enhanced data integrity, security, and sharing across disparate healthcare systems without compromising patient privacy.
Predictions for the Next Decade
Healthcare Data Management anticipates significant advancements in the integration of genomics and biometric data into everyday healthcare practices. This integration will enable truly personalized medicine, where treatment plans are tailored to the genetic profiles of individuals. Additionally, the Internet of Medical Things (IoMT) will likely become ubiquitous, with an array of connected devices providing continuous health monitoring and real-time data collection. This will not only improve patient outcomes but also transform the efficiency of healthcare delivery.
Preparing for Future Challenges
To leverage the benefits of these technologies fully, healthcare organizations must prepare to address several key challenges. These include managing the massive influx of data from diverse sources, ensuring compliance with evolving regulations, and safeguarding against new security vulnerabilities introduced by interconnected devices. Additionally, there is a pressing need for upskilling healthcare professionals to utilize advanced healthcare data management tools effectively, ensuring that technological advancements enhance rather than hinder the quality of care.
Case Studies and Success Stories
Innovative Healthcare Data Management Projects
One notable example is a project undertaken by a major hospital chain that implemented a predictive analytics tool using AI to identify patients at risk of readmission within 30 days of discharge. This tool analyzes historical patient data, current treatment plans, and post-discharge follow-up data to flag high-risk patients, enabling proactive interventions that have significantly reduced readmission rates and improved patient outcomes.
Lessons Learned from the Field
This case highlights several key lessons, including the importance of data quality and the need for a multidisciplinary approach to data management projects. Healthcare providers learned that success requires not only advanced technology but also strong collaboration between clinicians, data scientists, and IT specialists to ensure that the insights generated are actionable and clinically relevant.
Impact on Healthcare Delivery and Policy
The impact of effective healthcare data management extends beyond individual patient care to influence broader healthcare policies. For instance, comprehensive data analysis can help identify public health trends, inform policy decisions, and allocate resources more effectively. Additionally, success stories from data management initiatives often set new standards for healthcare practices, leading to widespread adoption of best practices and technological innovations across the healthcare sector.
Conclusion
Healthcare Data Management is a critical component that can significantly enhance patient outcomes and operational efficiencies in healthcare settings. As we look to the future, the integration of advanced technologies such as AI, blockchain, and cloud computing holds the promise of transforming healthcare data management by ensuring data security, improving interoperability, and facilitating real-time, data-driven decision-making. For healthcare organizations, embracing these technologies and adhering to rigorous data management practices will be key to achieving higher standards of patient care and navigating the complexities of modern healthcare systems.
FAQs on Healthcare Data Management
Q1: What is healthcare data management?
Healthcare data management involves the systematic organization, storage, and analysis of data created and collected in healthcare settings. It ensures that medical information is accurately maintained, easily accessible, and securely protected to support effective patient care and health service operations.
Q2: Why is data security important in healthcare?
Data security is crucial in healthcare to protect sensitive patient information from unauthorized access, breaches, and other cyber threats. It helps maintain patient trust and complies with legal requirements such as HIPAA, which mandates strict safeguards to ensure the confidentiality and integrity of health information.
Q3: How can artificial intelligence (AI) enhance healthcare data management?
AI can significantly improve healthcare data management by automating complex processes such as data entry, analysis, and reporting. It helps in predictive analytics, enabling healthcare providers to anticipate patient needs, personalize treatment plans, and improve outcomes based on data-driven insights.
Q4: What role does blockchain technology play in healthcare data management?
Blockchain technology offers a secure, transparent, and immutable ledger for managing healthcare data. It enhances data security, improves the traceability of medical records, and enables secure sharing of information across different healthcare providers, reducing the risk of data breaches and errors.
Q5: What are the future trends in healthcare data management?
Future trends in healthcare data management include the increased adoption of cloud-based solutions for flexible, scalable storage, and the integration of Internet of Medical Things (IoMT) devices for real-time patient monitoring and data collection. Additionally, advancements in AI and machine learning will continue to refine the analytics capabilities essential for personalized medicine and preventive healthcare strategies.